For as broad and difficult of a problem running a search engine is and how many competing interests are involved, when Matt Cutts was at Google they ran a pretty clean show. Some of what they did before the algorithms could catch up was of course fearmongering (e.g. if you sell links you might be promoting fake brain cancer solutions) but Google generally did a pretty good job with the balance between organic and paid search.
Early in search ads were clearly labeled, and then less so. Ad density was light, and then less so.
It appears as a somewhat regular set of compounded growth elements on the stock chart, but it is a series of decisions. What to measure, what to optimize, what to subsidize, and what to sacrifice.
Savvy publishers could ride whatever signals were over-counted (keyword repetition, links early on, focused link anchor text, keyword domains, etc.) and catch new tech waves (like blogging or select social media channels) to keep growing as the web evolved. In some cases what was once a signal of quality would later become an anomaly ... the thing that boosted your rank for years eventually started to suppress your rank as new signals were created and signals composed of ratios of other signals got folded into ranking and re-ranking.
Over time as organic growth became harder the money guys started to override the talent, like in 2019 when a Google yellow flag had the ads team promote the organic search and Chrome teams intentionally degrade user experience to drive increased search query volume:
“I think it is good for us to aspire to query growth and to aspire to more users. But I think we are getting too involved with ads for the good of the product and company.” - Googler Ben Gnomes
A healthy and sustainable ecosystem relies upon the players at the center operating a clean show.
If they decide not to, and eat the entire pie, things fall apart.
One set of short-term optimizations is another set of long-term failures.
The specificity of an eHow article gives it a good IR score, and AdSense pays for a thousand similar articles to be created, then the "optimized" ecosystem gets a shallow sameness, which requires creating new ranking signals.
In the last quarter, Q1 of 2025, it was the first time the Google partner network represented less than 10% of Google ad revenues in the history of the company.
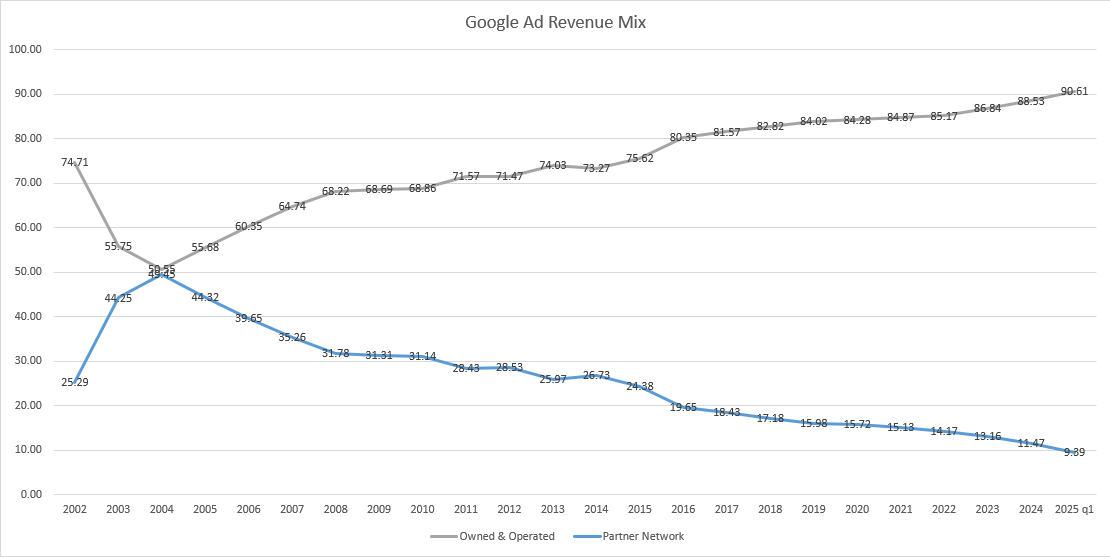
Google's fortunes have never been more misaligned with web publishers than they are today. This statement becomes more true each day that passes.
That ecosystem of partners is hundreds of thousands of publishers representing millions of employees. Each with their own costs and personal optimization decisions.
Publishers create feature works which are expensive, and then cross-subsidize the most expensive work with cheaper & more profitable works. They receive search traffic to some type of pages which are seemingly outperforming today and think that is a strategy which will help them into the future, though hitting the numbers today can mean missing them next year, as the ranking signal mix squeezes out profits from those "optimizations," and what led to higher traffic today becomes part of a negative sitewide classifier the lowers rankings across the board in the future.
Last August Googler Ryan Moulton published a graph of newspaper employees from 2010 until now, showing about a 70% decline. The 70% decline also doesn't factor in that many mastheads have been rolled up by private equity players which lever them up on debt and use all the remaining blood to pay interest payments - sometimes to themselves - while stiffing losses from the underfunded pension plans on other taxpayers.
The quality of the internet that we've enjoyed for the last 20 years was an overhand from when print journalism still made money. The market for professionally written text is now just really small, if it exists at all.
Ryan was asked "what do you believe is the real cause for the decline in search quality, then? Or do you think there hasn't been a decline?"
His now deleted response stated "It's complicated. I think it's both higher expectations and a declining internet. People expect a lot more from their search results than they used to, while the market for actually writing content has basically disappeared."
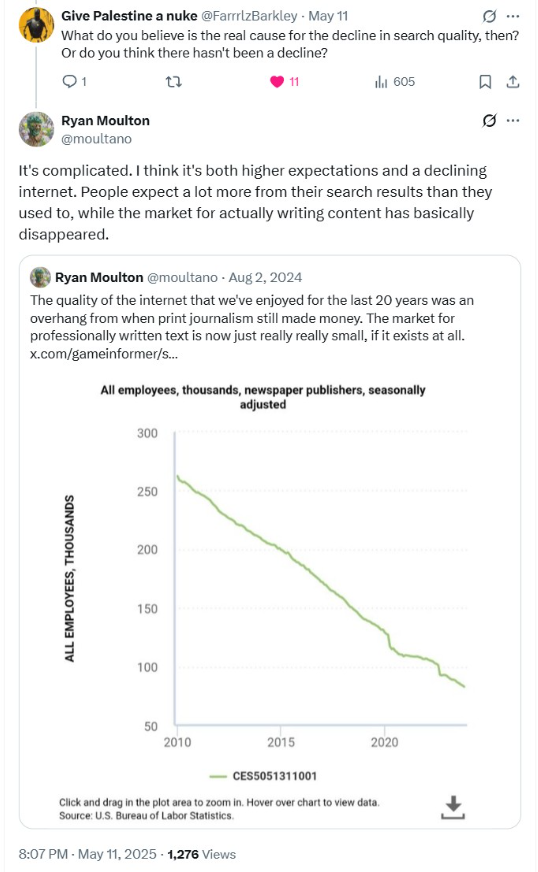
The above is the already baked cake we are starting from.
The cake were blogs were replaced with social feeds, newspapers got rolled up by private equity players, larger broad "authority" branded sites partner with money guys to paste on affiliate sections, while indy affiliate sites are buried ... the algorithmic artifacts of Google first promoting the funding of eHow, then responding to the success of entities like Demand Media with Vince, Panda, Penguin, and the Helpful Content Update.
The next layer of the icky blurry line is AI.
“We have 3 options: (1) Search doesn’t erode, (2) we lose Search traffic to Gemini, (3) we lose Search traffic to ChatGPT. (1) is preferred but the worst case is (3) so we should support (2)” - Google's Nick Fox
So long as Google survives, everything else is non-essential. ;)
AI overview distribution is up 116% over the past couple months.
Google features Reddit *a lot* in their search results. Other smaller forums, not so much. A company consisting of many forums recently saw a negative impact from algorithm updates earlier this year.
Going back to that whole bit about not fully disclosing economic incentives risks promoting brain cancer ... well how are AI search results constructed? How well do they cite their sources? And are the sources they cited also using AI to generate content?
"its gotten much worse in that "AI" is now, on many "search engines", replacing the first listings which obfuscates entirely where its alleged "answer" came from, and given that AI often "hallucinates", basically making things up to a degree that the output is either flawed or false, without attribution as to how it arrived at that statement, you've essentially destroyed what was "search." ... unlike paid search which at least in theory can be differentiated (assuming the search company is honest about what they're promoting for money) that is not possible when an alleged "AI" presents the claimed answers because both the direct references and who paid for promotion, if anyone is almost-always missing. This is, from my point of view anyway, extremely bad because if, for example, I want to learn about "total return swaps" who the source of the information might be is rather important -- there are people who are absolutely experts (e.g. Janet Tavakoli) and then there are those who are not. What did the "AI" response use and how accurate is its summary? I have no way to know yet the claimed "answer" is presented to me." - Karl Denninger
The eating of the ecosystem is so thorough Google now has money to invest in Saudi Arabian AI funds.
Periodically ViperChill highlights big media conglomerates which dominate the Google organic search results.
One of the strongest horizontal publishing plays online has been IAC. They've grown brands like Expedia, Match.com, Ticketmaster, Lending Tree, Vimeo, and HSN. They always show up in the big publishers dominating Google charts. In 2012 they bought About.com from the New York Times and broke About.com into vertical sites like The Spruce, Very Well, The Balance, TripSavvy, and Lifewire. They have some old sites like Investopedia from their 2013 ValueClick deal. And then they bought out the magazine publisher Meredith, which publishes titles like People, Better Homes and Gardens, Parents, and Travel + Leisure. What does their performance look like? Not particularly good!
DDM reported just 1% year-over-year growth in digital advertising revenue for the quarter. It posted $393.1 million in overall revenue, also up 1% YOY. DDM saw a 3% YOY decline in core user sessions, which caused a dip in programmatic ad revenue. Part of that downturn in user engagement was related to weakening referral traffic from search platforms. For example, DDM is starting to see Google Search’s AI Overviews eat into its traffic.
Google's early growth was organic through superior technology, then clever marketing via their toolbar, and later a set of forced bundlings on Android combined with payolla for default search placements in third party web browsers. A few years ago the UK government did a study which claimed if Microsoft gave Apple a 100% revshare on Bing they still couldn't compete with the Google bid for default search placement in Apple Safari.
Microsoft offered over a 100% ad revshare to set Bing as the default search engine and went so far as discussing selling Bing to Apple in 2018 - but Apple stuck with Google's deal.
In search, if you are not on Google you don't exist.
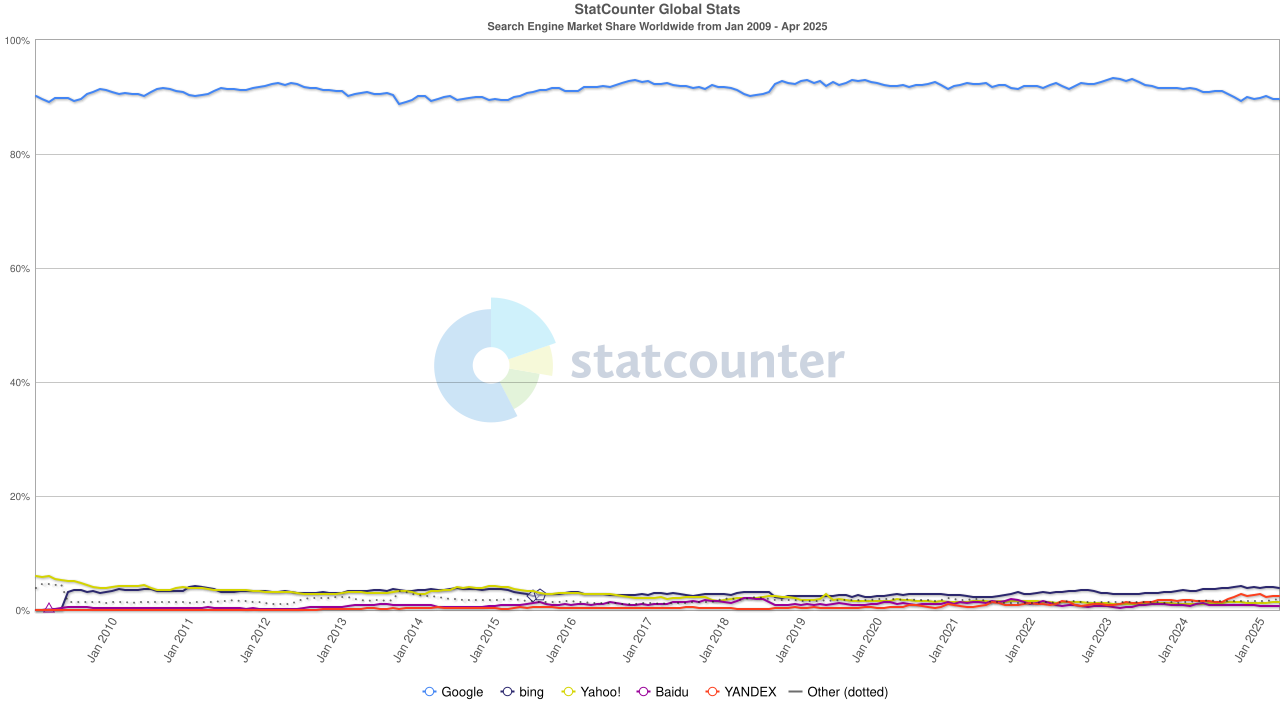
As Google grew out various verticals they also created ranking signals which in some cases were parasitical, or in other cases purely anticompetitive. To this day Google is facing billions in of dollars in new suits across Europe for their shopping search strategy.
The Obama administration was an extension of Google, so the FTC gave Google a pass in spite of discovering some clearly anticompetitive behavior with real consumer harm. The Wall Street Journal published a series of articles from getting half the pages of the FTC research into Google's conduct:
"Although Google originally sought to demote all comparison shopping websites, after Google raters provided negative feedback to such a widespread demotion, Google implemented the current iteration of its so-called 'diversity' algorithm."
What good is a rating panel if you get to keep re-asking the questions again in a slightly different way until you get the answer you want? And then place a lower quality clone front and center simply because it is associated with the home team?
"Google took unusual steps to "automatically boost the ranking of its own vertical properties above that of competitors,” the report said. “For example, where Google’s algorithms deemed a comparison shopping website relevant to a user’s query, Google automatically returned Google Product Search – above any rival comparison shopping websites. Similarly, when Google’s algorithms deemed local websites, such as Yelp or CitySearch, relevant to a user’s query, Google automatically returned Google Local at the top of the [search page].”"
The forced ranking of house properties is even worse when one recalls they were borrowing third party content without permission to populate those verticals.
Now with AI there is a blurry line of borrowing where many things are simply probabilistic. And, technically, Google could claim they sourced content from a third party which stole the original work or was a syndicator of it.
As Google kept eating the pie they repeatedly overrode user privacy to boost their ad income, while using privacy as an excuse to kneecap competing ad networks.
Remember the old FTC settlement over Google's violation of Safari browser cookies? That is the same Google which planned on depreciating third party cookies in Chrome and was even testing hiding user IP addresses so that other ad networks would be screwed. Better yet, online business might need to pay Google a subscription fee of some sort to efficiently filter through the fraud conducted in their web browser.
HTTPS everywhere was about blocking data leakage to other ad networks.
AMP was all about stopping header bidding. It gave preferential SERP placement in exchange for using a Google-only ad stack.
Even as Google was dumping tech costs on publishers, they were taking a huge rake of the ad revenue from the ad serving layer: "Google's own documents show that Google has siphoned off thirty-five cents of each advertising dollar that flows through Google's ad tech tools."
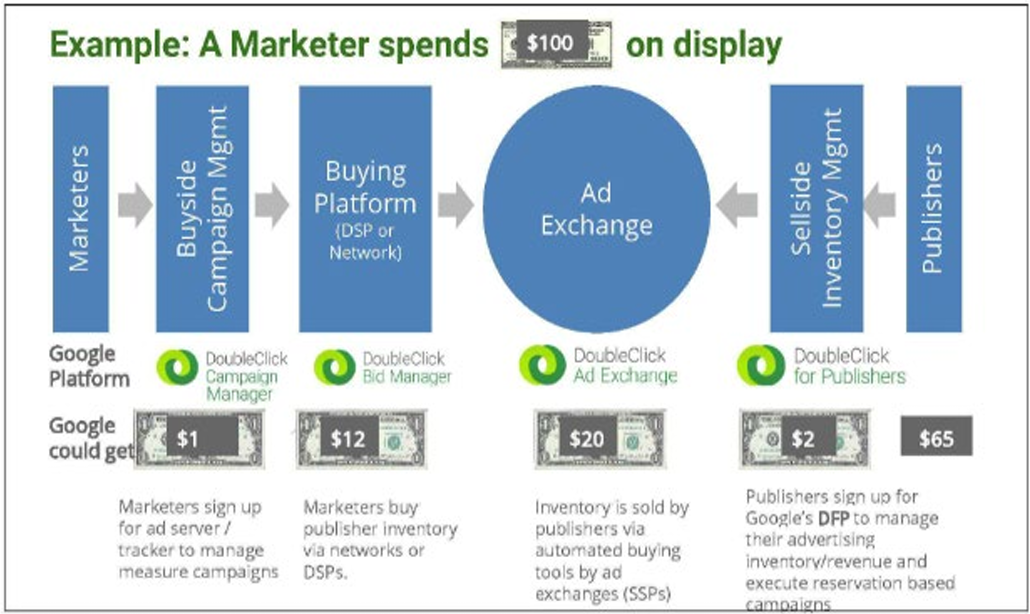
After acquiring DoubleClick to further monopolize the online ad market, Google merged user data for their own ad targeting, while hashing the data to block publishers from matching profiles:
"In 2016, as part of Project Narnia, Google changed that policy, combining all user data into a single user identification that proved invaluable to Google's efforts to build and maintain its monopoly across the ad tech industry. ... After the DoubleClick acquisition, Google "hashed" (i.e., masked) the user identifiers that publishers previously were able to share with other ad technology providers to improve internet user identification and tracking, impeding their ability to identify the best matches between advertisers and publisher inventory in the same way that Google Ads can. Of course, any puported concern about user privacy was purely pretextual; Google was more than happy to exploit its users' privacy when it furthered its own economic interests."
In terms of cost, I really don't think the O&O impact has been understood too, especially on YouTube. - Googler David Mitby
Did we tee up the real $ price tag of privacy? - Googler Sissie Hsiao
Google continues to spend billions settling privacy-related cases. Settling those suits out of court is better than having full discovery be used to generate a daisy chain of additional lawsuits.
As the Google lawsuits pile up, evidence of how they stacked the deck becomes more clear.